In our latest release, version 0.10.1.169, we now support Noisy-Nets as described by Fortunato et al.[1], Prioritized Experience Replay as described by Schaul et al.[2] and Deep Q-Learning reinforcement learning as described by Castro et al.[3] and The Dopamine Team[4] and use these to train MyCaffe to beat the ATARI game ‘Breakout’!
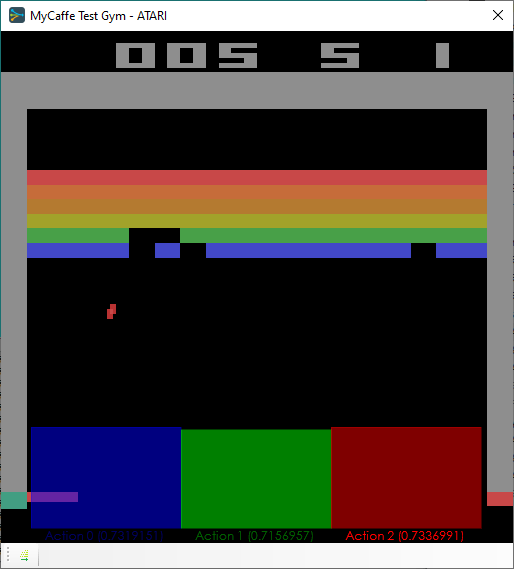
The action values are displayed in an overlay at the bottom of the ATARI Gym and updated during each step of the game.
The Noisy-Net model used with reinforcement learning is fairly simple, comprising of three CONVOLUTION layers followed by two INNERPRODUCT layers each of which have noise turned ON.
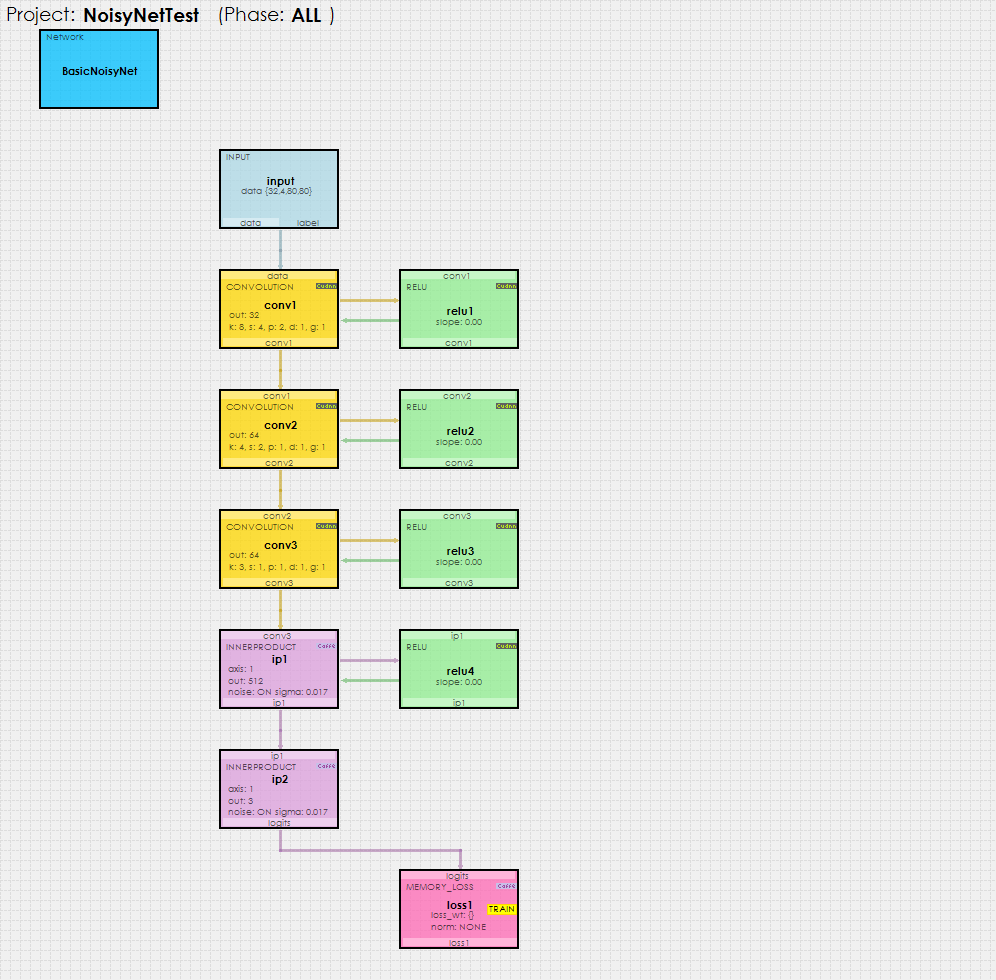
To try out this model and train it yourself, just check out our Tutorials for easy step-by-step instructions that will get you started quickly! For cool example videos, including a Cart-Pole balancing video, check out our Examples page.
New Features
The following new features have been added to this release
- CUDA 10.1.168/cuDNN 7.6.1/nvapi 410/driver 430.86 support added.
- Windows 1903 support added.
- Added Test support to RL trainers.
- Added TestMany support to RL trainers.
- Added DQN trainer support for Deep Q-Learning.
- Added ATARI breakout ROM.
- Added Noise support to InnerProduct layers for NoisyNets.
Bug Fixes
The following bug fixes have been made in this release.
- Fixed bugs in MemoryLoss layer.
- Fixed bugs in Convolution Editor, pad = 0 now accepted.
Happy Deep Learning!
[1] Meire Fortunato, Mohammad Gheshlaghi Azar, Bilal Piot, Jacob Menick, Ian Osband, Alex Graves, Vlad Mnih, Remi Munos, Demis Hassabis, Olivier Pietquin, Charles Blundell, Shane Legg, Noisy Networks for Exploration, arXiv:1706.10295, June 30, 2017.
[2] Tom Schaul, John Quan, Ioannis Antonoglou, David Silver, Prioritized Experience Replay, arXiv:1511.05952, November 18, 2015.
[3] Pablo Samuel Castro, Subhodeep Moitra, Carles Gelada, Saurabh Kumar, Marc G. Bellemare, Dopamine: A Research Framework for Deep Reinforcement Learning, arXiv:1812.06110, December 14, 2018.
[4] The Dopamine Team (Google), GitHub:Google/Dopamine, GitHub, Licensed under the Apache 2.0 License. Source code available on GitHub at google/dopamine.
[5] The Arcade Learning Environment: An Evaluation Platform for General Agents, by Marc G. Bellemare, Yavar Naddaf, Joel Veness and Michael Bowling, 2012-2013. Source code available on GitHub at mgbellemare/Arcade-Learning-Environment.
[6] Stella – A multi-platform Atari 2600 VCS emulator by Bradford W. Mott, Stephen Anthony and The Stella Team, 1995-2018. Source code available on GitHub at stella-emu/stella